Predictive analytics, a realm of data-driven insights and forward-thinking decisions, has earned its place as a cornerstone of modern business strategies. Yet, even within the glittering realm of predictive prowess, skepticism and criticism have found their foothold.
As your organization increasingly relies on this practice to drive their future endeavors, it becomes imperative to examine the flip side of the coin. In this exploration, we uncover the voices of dissent and the key criticisms leveled against predictive analytics.
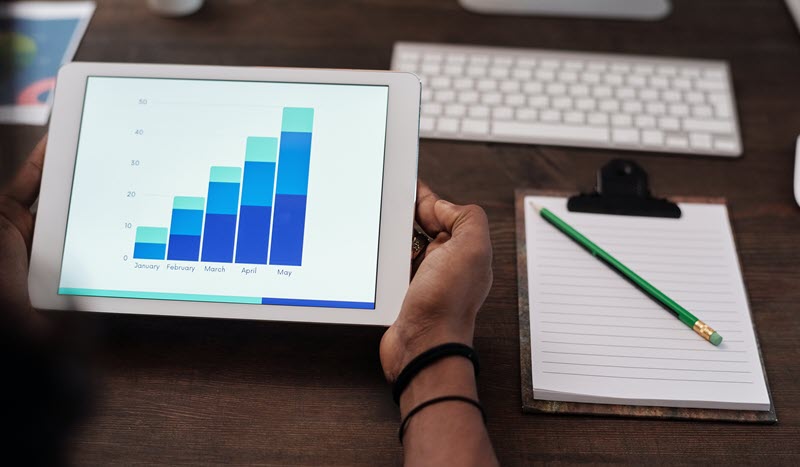
Major Points to Consider About Predictive Analytics
1. Data Quality and Bias
One of the most persistent critiques directed towards predictive analytics revolves around data quality and bias. Critics argue that the models built upon historical data can inherit the biases present in that data.
This bias can lead to skewed predictions that perpetuate existing disparities and inequalities. For instance, if historical data reflects discriminatory practices, predictive models may unintentionally perpetuate these biases, resulting in unfair outcomes.
2. Lack of Contextual Understanding
Predictive models excel at identifying correlations within datasets, but they often lack a contextual understanding of the underlying factors. Critics argue that predictions derived purely from correlations may overlook essential causal relationships. This can lead to misguided decision-making if your organization fails to recognize the true drivers behind the patterns.
3. Black Box Phenomenon
Some critics view predictive models as enigmatic “black boxes.” These models generate predictions, but their inner workings can be complex and difficult to decipher. This opacity raises concerns about accountability, interpretability, and the potential for unintended consequences. When models cannot be easily explained or understood, it becomes challenging to validate their accuracy or to assess potential biases.
4. Overfitting and Generalization
Predictive models trained on historical data can sometimes suffer from overfitting, a phenomenon where a model becomes too tailored to the training data. While the model performs exceptionally well on historical data, it might struggle to generalize to new, unseen data. This can lead to predictions that are overly optimistic and fail to accurately reflect real-world outcomes.
5. Rapidly Changing Environments
Critics highlight that predictive analytics might falter in rapidly changing environments. Historical data might not capture unprecedented events or unforeseen disruptions. In situations like the COVID-19 pandemic, where the past fails to predict the future, predictive models can struggle to provide meaningful insights.
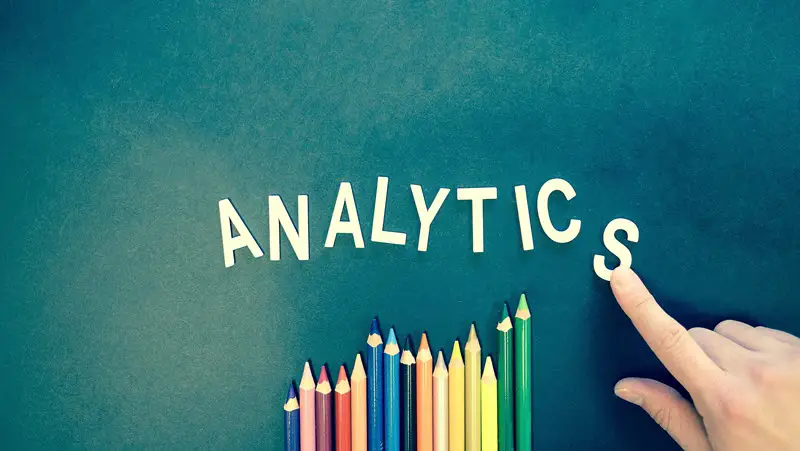
6. Dependency on Historical Patterns
Predictive analytics heavily relies on historical patterns to make predictions. Critics argue that this approach might not account for paradigm shifts, innovations, or evolving consumer behavior. Your organization can risk missing out on opportunities by placing too much faith in historical data.
7. Ignoring Human Expertise
Over reliance on predictive models can lead to the dismissal of human expertise. Critics contend that while data-driven insights are valuable, they should be considered alongside domain knowledge and human intuition. Disregarding human expertise can result in decisions that lack nuance and context.
8. Privacy and Ethical Concerns
Predictive analytics often involve analyzing personal data, raising concerns about privacy and ethical implications. Critics worry about the potential for data breaches, misuse of personal information, and the erosion of individual privacy rights. Your business must navigate these concerns carefully to maintain public trust.
Navigating the Criticisms
It’s important to view these concerns through a constructive lens. Addressing these criticisms involves a multi-faceted approach:
- Transparent Modeling: You can strive for transparent modeling, making the inner workings of predictive algorithms more understandable and interpretable.
- Ethical Considerations: Adhering to ethical guidelines and ensuring data privacy can alleviate concerns related to data misuse and breaches.
- Human-Machine Collaboration: A balanced approach involves combining data insights with human expertise, acknowledging that predictive analytics is a tool to augment decision-making rather than replace it.
- Regular Validation: Regularly validating and updating models with new data can help address concerns related to rapidly changing environments and overfitting.
So, while predictive analytics has revolutionized decision-making, it’s essential to embrace a critical perspective. Recognizing the limitations and potential pitfalls of predictive models is an important step towards harnessing their benefits effectively.
By addressing these criticisms and refining methodologies, your organization can navigate the path of predictive analytics with vigilance, ensuring that data-driven insights align with ethical standards, human expertise, and a comprehensive understanding of the complex landscape they seek to illuminate.